Replay at https://www.youtube.com/YouthAILab
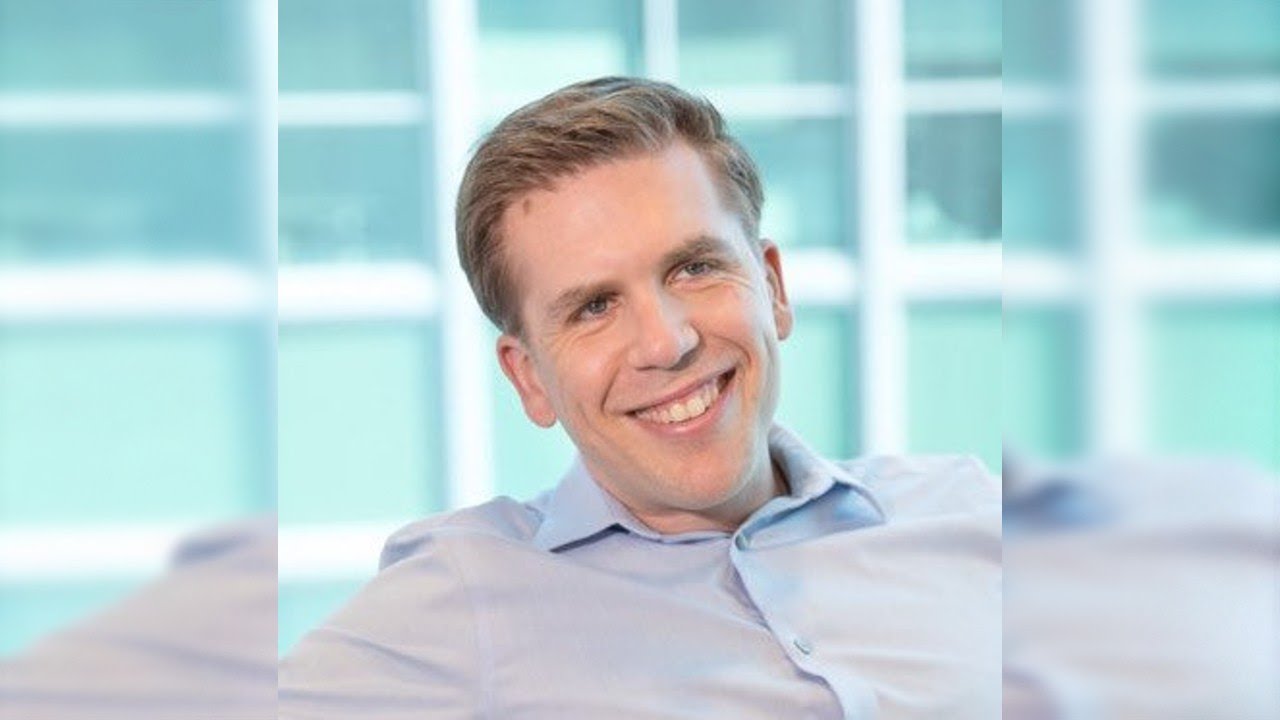
Full Summary:
Starting in electrical engineering before moving towards artificial intelligence, Dr. Leo Grady received his Ph.D. in computer vision, specializing in cognitive and neural systems. A few of his involvement in AI include Siemens, which developed AI applications in radiology and diagnostics, HeartFlow, which focused on cardiovascular assessment, and Paige, a clinical-grade AI in pathology with a complete software enterprise imaging platform.
Grady first mentions the other aspects involved in technology in healthcare. The increasing demand for healthcare because of the increasing age population is an example of the societal challenge of healthcare. With an increasing cost of healthcare, the question of innovation comes in the form of how the demand for healthcare can be fulfilled at a lower price. Therefore, leading to his point of AI’s triple aim: to improve health, to lower costs, and to provide better care.
Bottom line, AI is a technology that enables complex pattern recognition and action, meaning in healthcare, it isn’t a robot doctor. In fact, AI doesn’t appear as a robot frequently, often appearing in the postal system, search engines, media recommendations, or facial recognition.
A clear example of this is HeartFlow, which takes and CT scan of someone’s heart and uses AI to find specific blood vessels, plaque or blockages, and the lumen surface. Through this technology and computational three-dimensional model, HeartFlow calculates the blood flow through arteries. Its implementation is less invasive, allowing doctors to know whether a patient could have a heart attack using this measurement of blood pressure. Grady also mentions Paige, the first FDA-approved AI system in pathology, which involves using AI innovation to assist pathologists to diagnose cancer.
AI in healthcare, according to Grady, can be split into four different categories: automate or support for diagnosis, automate manually intensive tasks at a large scale, enable technology for new medical devices, and enable technology that performs newfound tasks, which could replace existing hardware.
The concept of an “AI robot” is similar to an aid or tool for more accuracy and efficiency, which supports an existing diagnosis or can diagnose problems in health. Companies that provide this technology include Paige, Iterative Scopes, Cleerly, Arterys, Philips, IBM Watson Health, and Siemens Healthineers. Automated, manual-intensive AI are systems, like those from Atropos Health, Suki, or Soley Therapeutics, that can do manual work, allowing doctors to work faster and divert attention to other tasks. Its use comes with its speed and the scale at which it interprets data, useful for research. Examples of AI technology in new medical devices include Subtle Medical, Butterfly Medical, Intuitive Surgical, inHEART, MediVis, and Cionic. These companies have technology that uses AI, which can be more cost-effective and efficient. HeartFlow and Paige are both examples of AI that perform newfound tasks. Another of which is Neuralight, which applies computer vision that uses eye tracking to detect Parkinson’s and Alzheimer’s. AI-central companies do not automate or support tasks and diagnoses, but rather use AI to “go beyond.”
Data is the blood of AI; in healthcare, it can be taken from a healthcare provider, by providing a service where data passes through, directly from the consumer like an Apple Watch, or from the public domain. The challenge of data in healthcare comes from data privacy and security because of present laws and sensitivity towards the data. In the United States, for instance, HIPAA enforces regulations that forbid the disclosure of identifiable information of a person, like social security numbers or names; in Europe, similarly, the GDPR requires companies to provide privacy capabilities. Security is also important: without security, data and information can be leaked even with a heavy emphasis on privacy. Therefore, many companies use third parties like ISO27001, AICPA SOC 2, or FedRamp to regulate security.
Critics believe that AI is overhyped or too dangerous, writing media that argue AI doesn’t work as well as advertised. A significant concern is a bias in AI—especially an issue with racial, socio-economic, and gender differences in data— due to poor validation of data, like overfitting. Biases are recognized in healthcare, even beyond AI, as seen with how drugs tested more in men may affect women taking the prescribed end product differently. Therefore, there are more precautions in the FDA to prevent such biased sampling.
As for marketing and pricing AI, the perceived value of the product matters for consumers to buy or pass on it. The factors of perceived value include actual value, product scarcity, urgency and demand, company brand versus value brand, and testimonials from trusted customers. An example Grady provides is how cancer treatments cannot be expected as a few cents or dollars, unlike band-aids.
Pricing requires looking at the value of similar products in comparison. In HeartFlow, the technology can be seen either as low in cost or high in cost depending on the way it’s marketed. Calling the product an algorithm used for post-processing CT scans versus calling it a replacement for cath lab procedures brings in major, major profit differences. CT scans are often covered by insurance or low cost, while cath lab procedures cost thousands and thousands. This only reiterates the importance of how a product is marketed and displayed.
Grady emphasizes that there is more to AI technology than just simple AI. User experience, product design, regulations, marketing, pricing, and product support are only some of the many factors that come into play for AI in healthcare. He concludes with the importance of understanding the different aspects of AI, considering the parts that make AI and other products successful in consumer applications.
For previous Youth AI talks, visit https://www.youtube.com/YouthAILab
0 Comments